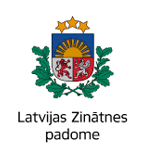
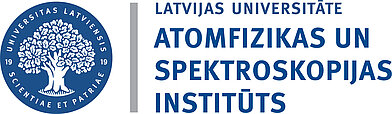
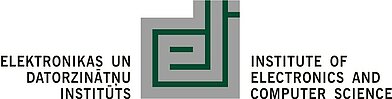
Project title: Histological recognition and analysis of veterinary tumors surgical margins by using artificial intelligence and multimodal imaging
Project number: lzp-2022/1-0274
Project applicant: University of Latvia (UL)
Project cooperation partner: Institute of Electronics and Computer Science (IECS)
Research manager: Dr. phys. Mindaugas Tamosiunas
Project implementation deadline: 01.01.2023. – 31.12.2025.
Total funding of the project: EUR 300,000, including the funding allocated to the UL part of the project 195 622,00 EUR and the part of the IECS 104 378,00 EUR
Planned project results:
· original scientific articles the quoting index whereof reaches at least 50 per cent of the average quoting index of the sector which have been submitted or accepted for publication in the journals or conference symposia included in Web of Science Core Collection or SCOPUS databases – 3
· original scientific articles submitted or accepted for publication in the journals or conference symposia included in Web of Science Core Collection or SCOPUS databases – 3
· scientific databases and data sets developed within the scope of the project – 1
· functional models – 1
· new non-commercial treatment and diagnostic methods – 1
· project proposal submitted in an international or national call for research and development projects – 2
· successfully defended master’s thesis within the thematic focus of the project – 2
· doctoral thesis successfully defended according to certain procedures within the thematic focus of the project – 1
Project Summary:
The project will introduce new optical methods into veterinary oncology, significantly simplifying histological sample preparation and allowing veterinarians to receive histopathology (HP) results in near real-time. Currently, standard HP analysis takes several days and can screen only a limited area for clean surgical margins. The implemented method will allow label-free HP-type tumor analysis and recognition of clean surgery margins for the most frequent canine and feline tumors like soft tissue sarcomas and mast cell tumors. Our proposed innovation addresses autofluorescence imaging, Raman spectral band imaging and optical coherence tomography (OCT) implementation into the tissue histological pattern recognition by generative adversarial networks (GAN). Previously, we showed that a Raman-OCT multimodal strategy with machine learning outperformed the existing clinical methods like fine-needle aspiration by separating malignant and benign tissues with >90% sensitivity and specificity. The proposed label-free multimodal imaging with GAN implementation will improve the automated histological analysis of unstained tissues ex vivo, allowing microscope level guidance to identify the incomplete histologic margins. The team experience will allow embedding the multimodal analysis setup and GAN algorithms into a prototype device available in Latvian veterinary oncology clinics.